Unleashing the transformative role of Artificial Intelligence in tuberculosis control
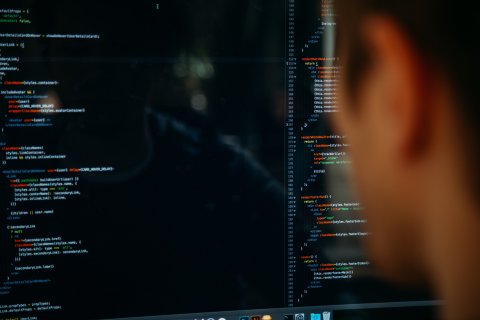
Dr Aparna Sen Chaudhary, Associate Programme Manager, Wadhwani AI, explains how AI in machine learning and natural language has revolutionised our approach to infectious disease detection, prevention, and treatment, providing a potent tool to combat field challenges.
Infectious diseases have constantly threatened human health and well-being throughout history. From the Black Death in the Middle Ages to the more recent COVID-19 pandemic, these diseases have wreaked havoc, remaining the primary cause for the majority of mortality, morbidity and loss of productivity, especially in low and middle-income countries.
Although most infectious diseases affecting humans have some detection and treatment options available, the cost and lack of efficacy, coupled with the rapid development of drug resistance in a resource-constraint environment, have resulted in unsatisfactory progress in preventing and controlling these diseases.
Artificial Intelligence (AI) in machine learning and natural language has revolutionised our approach to infectious disease detection, prevention, and treatment, providing a potent tool to combat field challenges.
Tuberculosis (TB), an infectious disease, has remained one of the leading causes of morbidity and mortality. For decades, TB has been a global health challenge and through its End TB Strategy, the World Health Organization (WHO) has aimed to end the TB epidemic by reducing TB-related deaths by 95% and by cutting new cases detected by 90% by 2035.
On a similar line, the Government of India, under its National Strategic Plan (NSP) 2017-2025, the National Tuberculosis Elimination Programme (NTEP) has planned to reduce TB deaths by 90% and reduce TB incidence by 80% by 2025 from the baseline of 2015, five years ahead of global timelines set for the country.
Globally, many TB patients go unreported yearly; in 2021 alone, there were approximately 3.7 million missed cases (i.e., the gap between the estimated number and the diagnosed and reported cases). India alone contributes to roughly 0.5 million, per the India TB Report 2020.
One of the primary reasons for this is the limited accessibility and availability of an economical screening tool that is easy to use at the point of care, especially in low and middle-income healthcare settings.
To curtail this, in 2021, in its Tuberculosis guidelines, WHO approved the use of artificial intelligence for TB detection. Along similar lines, currently in India, there are two AI-based tools, namely artificial-intelligence-based computer-aided detection (AI-CAD) to analyse chest X-rays for tuberculosis, and AI-aided tool using cough sounds of different intensities to detect tuberculosis at an early stage, are being explored by the government in the public health field settings to assess their feasibility in reduce the “missed” TB cases gap within resource constraint settings.
In addition, TB mortality still poses a considerable challenge globally. A total of 1.6 million people were reported to have died from TB in 2021 despite the availability of quality-assured TB diagnosis and treatment services. Being a curable disease, the mortality from TB is approximately 50%, without treatment or with interrupted treatment. Poor adherence to treatment is a known causal factor for increased risk of morbidity, mortality and cost burden.3 Despite all efforts towards TB control, more than 4% of the diagnosed TB patients fail to adhere to their treatment (Global TB Report, 2022). Approximately 3% of TB patients in India are reported as non-adherent to TB treatment (India TB Report, 2022). Such cases significantly affect the successful implementation of TB control measures as they spread the disease within the community and often relapse as a severe form of TB.
Global studies
A few global studies have explored the use of the AI approach to predict TB patients at risk for treatment non-adherence and poor prognosis of TB.4 Presently, in India, under the guidance of the Health Ministry, an indigenous AI tool is developed which predicts the patient at risk of treatment non-adherence and mortality for the TB patients started on treatment. Thus aiding the field healthcare staff in triaging the patients requiring intensive support in a limited-resource setting, eventually reducing the burden of non-adherence and mortality.
Likewise, drug-resistant TB (DR-TB) is also a significant threat to the “End TB Strategy” target of reducing TB-related deaths by 95% by 2035. As per the Global TB Report 2022, there were an estimated 0.5 million cases of DR-TB in 2021, wherein approximately 20% were reported with pre-XDR (i.e., resistance to any fluoroquinolone). The number was projected to be approximately 0.1 million in India for the same year (India TB Report, 2023).
Under the National Tuberculosis Elimination Programme, Line Probe Assay (a rapid testing technique based on Polymerase chain reaction used to determine the drug-resistance profile for Mycobacterium tuberculosis) is used for diagnosing DR-TB among all microbiologically confirmed TB patients, which is estimated to be approximately one million. However, the test results often have prolonged turn-around time and errors in interpretation due to the high number of tests being performed in resource constraint settings, thus resulting in treatment initiation delay among DR-TB cases. Therefore, it was critical for the country to explore the value of AI to assist in the rapid interpretation of drug resistance profiles directly from LPA strips in place of manual interpretation. The AI tool allows almost real-time diagnosis of drug resistance cost-effectively, along with reducing the turn-around time and errors compared to the traditional approaches.
Ethical considerations
Ethical considerations are meticulously maintained in the healthcare sector by adhering to strict AI principles and practices. Patient data privacy and security are paramount, with robust encryption and consent mechanisms in place. Transparent AI algorithms are employed, ensuring that both healthcare providers and patients can comprehend and trust the decisions made by AI systems. Continuous monitoring and assessment are conducted to detect and mitigate biases, ensuring equitable care for all. Human oversight remains essential, with healthcare professionals empowered to override AI recommendations when needed. These ethical safeguards prioritise patient well-being and autonomy and guarantee responsible AI deployment in healthcare, upholding the highest standards of ethics and patient care.
Thus, AI has emerged as a transformative force in the battle against infectious diseases. Its ability to process vast amounts of data quickly and accurately, predict outbreaks, accelerate drug discovery, enhance diagnostic accuracy, and personalise treatment strategies has revolutionised infectious disease management in recent years. AI has emerged as a powerful ally in TB control efforts by assisting in early detection, drug resistance predictions, and treatment adherence. By harnessing the potential of AI, we can make significant strides in our mission to eradicate this deadly disease.
References
- World Health Organization. WHO consolidated guidelines on tuberculosis. Module 2: screening-systematic screening for tuberculosis disease. World Health Organization; 2021 Mar 22.
- Wadhwani AI. Tuberculosis. India.
- Pradipta IS, Houtsma D, van Boven JF, Alffenaar JW, Hak E. Interventions to improve medication adherence in tuberculosis patients: a systematic review of randomised controlled studies. NPJ primary care respiratory medicine. 2020 May 11;30(1):21.
- Gichuhi HW, Magumba M, Kumar M, Mayega RW. A machine learning approach to explore individual risk factors for tuberculosis treatment non-adherence in Mukono district. PLOS Global Public Health. 2023 Jul 3;3(7):e0001466.